The Role of p-Value in Inferential Tests
Inferential tests play a crucial role in determining the likelihood of observed data under the assumption that the null hypothesis (H0H_0H0) is true. This likelihood is expressed through the p-value, which quantifies how often we would expect the obtained results to occur purely by chance. In statistics, a result is deemed statistically significant when it is unlikely to have occurred by chance alone, based on a pre-established cutoff known as the significance level, denoted as α\alphaα. The most commonly used significance level is 0.05 or 5%. This means that if the p-value is less than α\alphaα, the data provides sufficient evidence to reject the null hypothesis and accept the alternative hypothesis (HaH_aHa).
For example, a p-value less than 0.05 suggests that the likelihood of obtaining the observed results, assuming the null hypothesis is true, is less than 5%. This indicates that the findings are rare or surprising enough to warrant rejecting the null hypothesis. On the other hand, if the p-value is greater than α\alphaα, the evidence is not strong enough to reject the null hypothesis, meaning we do not accept the alternative hypothesis.
Interpreting the p-Value and Type I Error
The p-value also represents the Type I Error Rate, which is the probability of incorrectly rejecting the null hypothesis when it is actually true. For instance, if the p-value is 0.05, it indicates a 5% chance of making a Type I Error. Lower p-values, such as 0.01, reflect even greater confidence in rejecting the null hypothesis, as the likelihood of error is reduced.
Returning to the example of the relationship between depression and smoking among daily young adult smokers, the p-value was calculated as 0.17. Since this is greater than 0.05, the data does not provide enough evidence to reject the null hypothesis. This means we cannot conclude that there is an association between depression and the number of cigarettes smoked per day in this population. Instead, the null hypothesis is accepted, indicating no significant relationship between smoking and depression in this case.
Modifying the Research Question: Broader Population and Revised Findings
Changing the parameters of the research question can lead to different findings. In a revised scenario, the analysis expanded to include all young adults who smoked in the past year, not just daily smokers. This adjustment increased the sample size to 1,706 individuals. The results showed that young adults with depression smoked an average of 351.7 cigarettes per month with a standard deviation of 300, while those without depression smoked 313.5 cigarettes per month with a standard deviation of 268.2. The difference of 38.2 cigarettes per month, equivalent to nearly two packs, yielded a p-value of 0.0285, which is less than the significance level of 0.05.
In this scenario, the p-value indicates that the probability of observing this difference by chance, assuming the null hypothesis is true, is less than 3%. Consequently, the null hypothesis is rejected, and it is concluded that young adults with depression smoke significantly more cigarettes per month than those without depression. This finding suggests a significant association between smoking and depression in this broader population.
Confidence in Findings and Implications
The lower p-value in the revised analysis provides a stronger level of certainty. With a p-value of 0.0285, the probability of making a Type I Error—wrongly rejecting the null hypothesis—is less than 3%. This means that if the study were repeated with similar sampling, the conclusion would be correct more than 97% of the time. Such a level of confidence aligns with scientific standards for declaring a significant association, reinforcing the conclusion that there is a meaningful relationship between smoking and depression among young adults who smoked in the past year.
This example highlights the importance of defining research parameters carefully, as changes in sample selection and variable scope can significantly influence findings and their interpretation. It also underscores the role of the p-value in guiding decisions about hypothesis testing and the confidence researchers can place in their conclusions.
More Articles
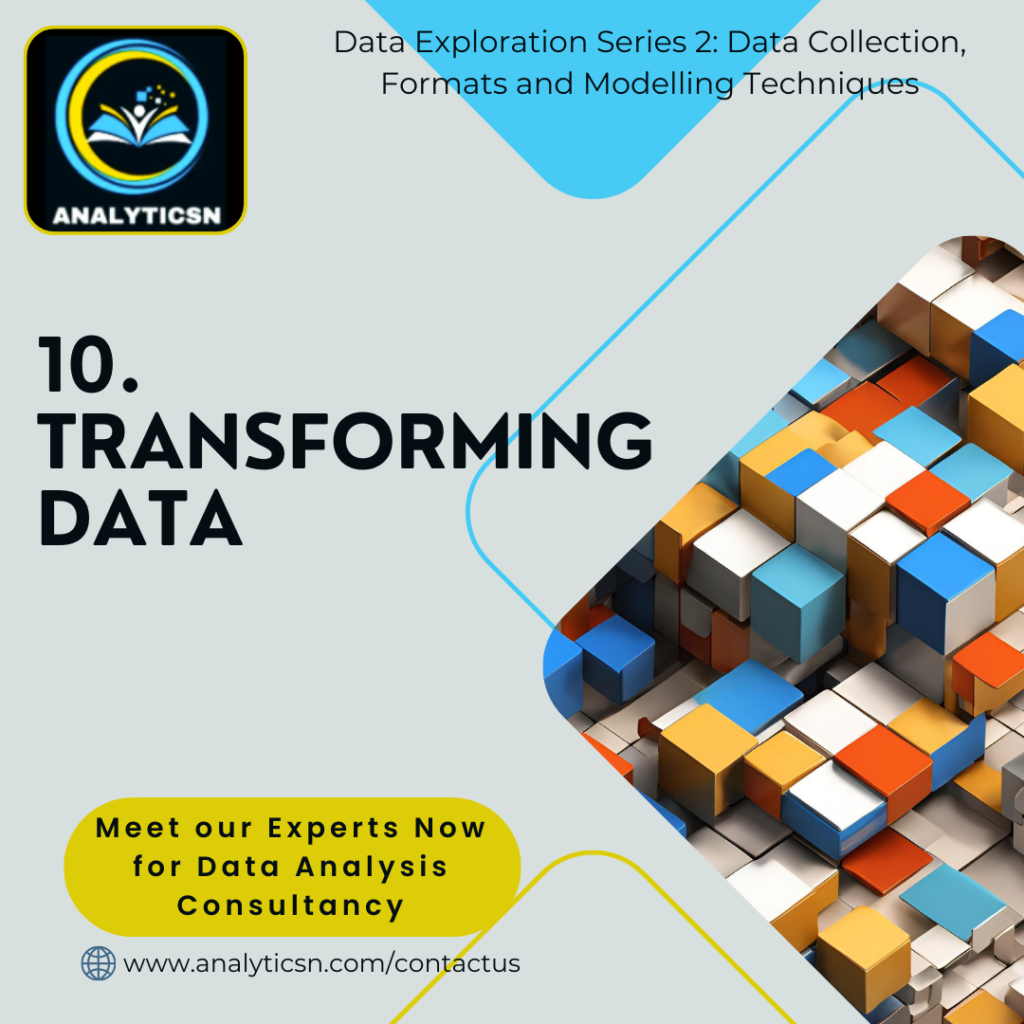
10. A Secret to Data Transformation
What is data transformation? A woman presenting data, a hand holding a medal, two people chatting, a ship’s wheel being...
Learn More >