Introduction to Hypothesis Testing
Hypothesis testing is a fundamental tool in inferential statistics, used to make decisions about populations based on sample data. It involves assessing whether there is sufficient evidence in the data to support or refute a hypothesis about a population parameter. This process is crucial in real-world applications where direct examination of entire populations is impractical. Various statistical tests, such as the Analysis of Variance (ANOVA) and the Chi-Square Test of Independence, are employed in hypothesis testing, but they all follow the same essential steps. These steps include specifying the null and alternative hypotheses, choosing a sample, assessing evidence, and drawing conclusions.
Step 1: Formulating Hypotheses
The first step in hypothesis testing is to define the null hypothesis (H0H_0H0) and the alternative hypothesis (HaH_aHa). The null hypothesis typically states that there is no effect or no difference in the parameter of interest. In contrast, the alternative hypothesis posits that there is an effect or difference. For example, consider the relationship between depression and smoking behavior. The null hypothesis states that there is no difference in smoking quantity between individuals with and without depression. The alternative hypothesis suggests that there is a difference, which could manifest as smokers with depression either consuming more or fewer cigarettes than those without depression.
Step 2: Selecting a Sample
The next step involves selecting a sample from the population to test the hypotheses. In this example, data from the NESARC dataset, a representative sample of 43,093 U.S. adults, is used. To focus the analysis, the sample is narrowed down to young adults aged 18–25 who are daily smokers, resulting in a subset of 1,320 individuals. Among this group, individuals with depression smoked an average of 13.9 cigarettes per day with a standard deviation of 9.2, while those without depression smoked an average of 13.2 cigarettes per day with a standard deviation of 8.5. While the average for those with depression is slightly higher, this observed difference may not be significant enough to reject the null hypothesis.
Step 3: Assessing the Evidence
This step involves evaluating the data to determine whether the observed difference between groups is significant or could have occurred by chance. The key question is whether the difference of 0.7 cigarettes per day between smokers with and without depression is sufficiently unusual under the assumption that the null hypothesis is true. This is assessed by calculating the probability of observing such a difference due to random variation alone, a measure known as the p-value.
In this example, the probability of observing a difference of this magnitude or greater, assuming the null hypothesis is true, is approximately 0.17 (17%). This means that if we repeatedly took random samples from the population, about 17 out of 100 samples would show a difference of 0.7 cigarettes per day purely by chance.
Step 4: Making a Decision
The final step is deciding whether to reject or fail to reject the null hypothesis based on the calculated probability. A p-value of 0.17 indicates a relatively high chance of observing the difference by random variation, which weakens the evidence against the null hypothesis. In hypothesis testing, the threshold for rejecting the null hypothesis, known as the significance level (α\alphaα), is often set at 0.05 (5%). This means that a p-value below 0.05 would lead to rejecting the null hypothesis, while a p-value above 0.05 would result in failing to reject it.
In this case, with a p-value of 0.17, there is insufficient evidence to confidently reject the null hypothesis. This means that while the data suggest a difference in smoking quantity, it is not statistically significant at the 5% level. Researchers must also consider the trade-offs involved in making errors. A higher p-value, such as 0.50, would suggest complete uncertainty akin to flipping a coin, while a smaller p-value, such as 0.05 or 0.01, provides stronger confidence in rejecting the null hypothesis.
Conclusion and Guidelines for Decision-Making
Hypothesis testing is a structured process that helps quantify uncertainty and make informed decisions about population parameters. The decision to reject or fail to reject the null hypothesis hinges on the p-value and its comparison with a predetermined significance level. While a p-value of 0.17 might not be compelling enough to reject the null hypothesis, lower probabilities, such as 0.05 or 0.01, provide stronger grounds for making such decisions. Establishing clear thresholds and understanding the implications of errors are essential for drawing reliable conclusions in statistical research.
More Articles
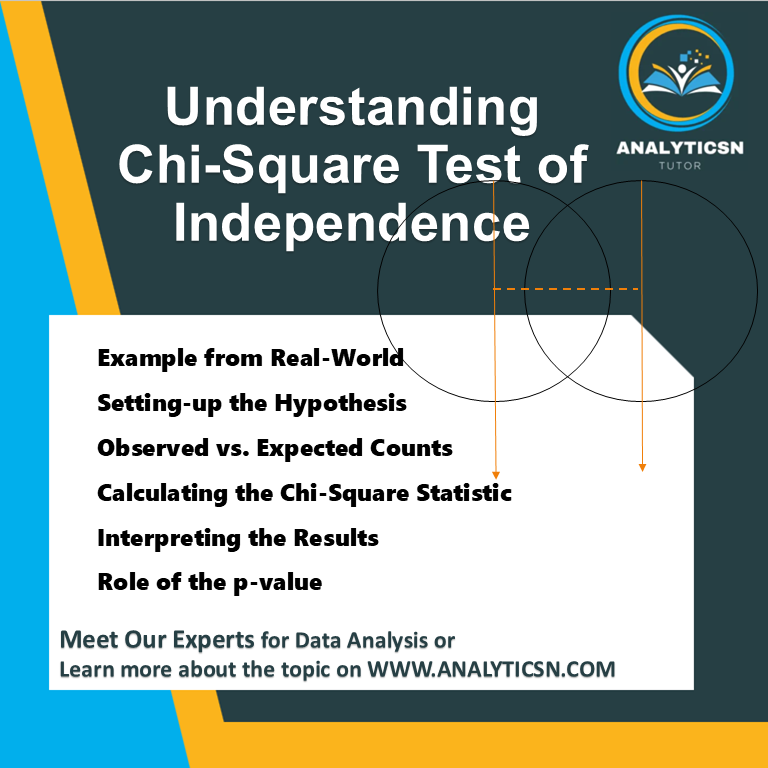
Need to Understand the Chi-Square Test of Independence
The Chi-Square Test of Independence is a statistical tool used to evaluate relationships between two categorical variables. Unlike Analysis of...
Learn More >